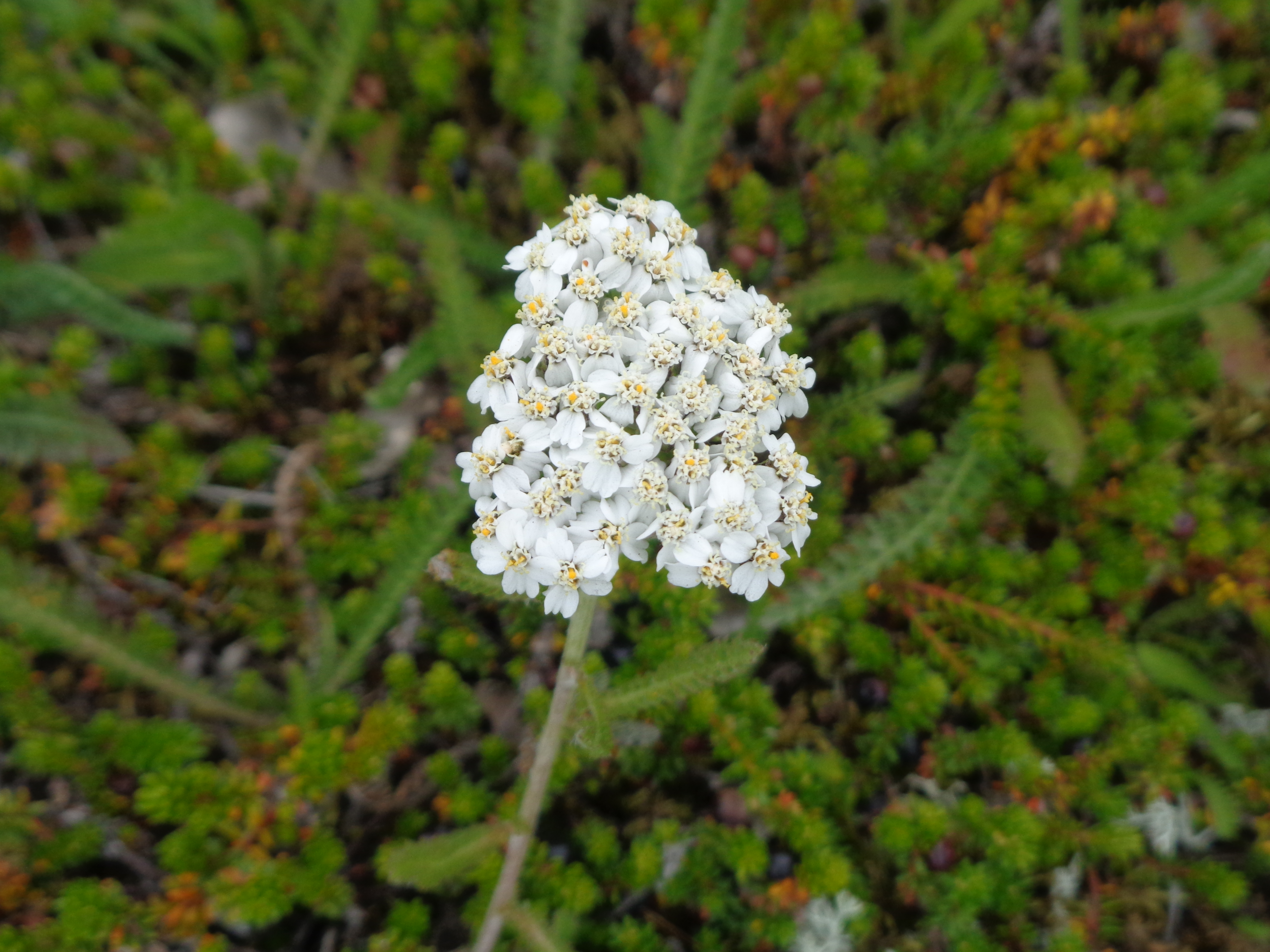
By design or necessity, contemporary optimization models, ranging from small problems in system control through classical nonlinear and semidefinite programs to huge examples in high-dimensional statistics, image processing, and machine learning, must confront the challenge of nonsmoothness. In practice, however, a typical geometric picture emerges, blending sharp and smooth behavior, and underlying algorithm analysis and design. This talk explains this phenomenon, and illustrates with algorithms like quasi-Newton, prox-linear, and primal-dual splitting methods, applied to examples from eigenvalue optimization and signal reconstruction.
Joint work with J. Burke (Washington), D. Drusvyatskiy (Washington), J. Guo (Cornell), D. Henrion (Toulouse), A. Ioffe (Technion), J. Liang (Cambridge), M. Overton, (Courant), S. Wright (Wisconsin)